Articles
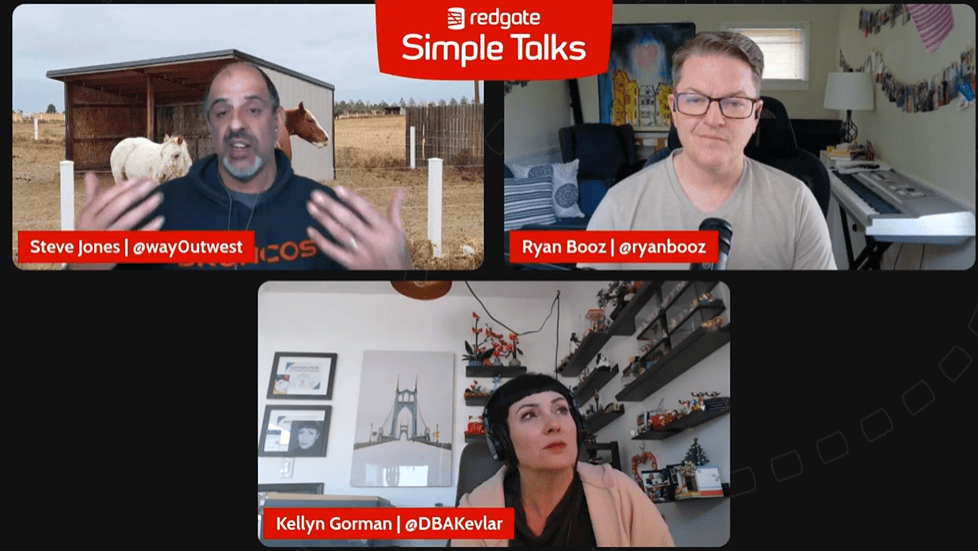
Redgate Advocates: Job Search Advice
Subscribe to the Simple Talk newsletter
Focus your database learning
Visit a curated selection of database-specific events and resources
Is your preferred database not listed?
As this is a growing list, contact us to let us know what you’d like to see added next.
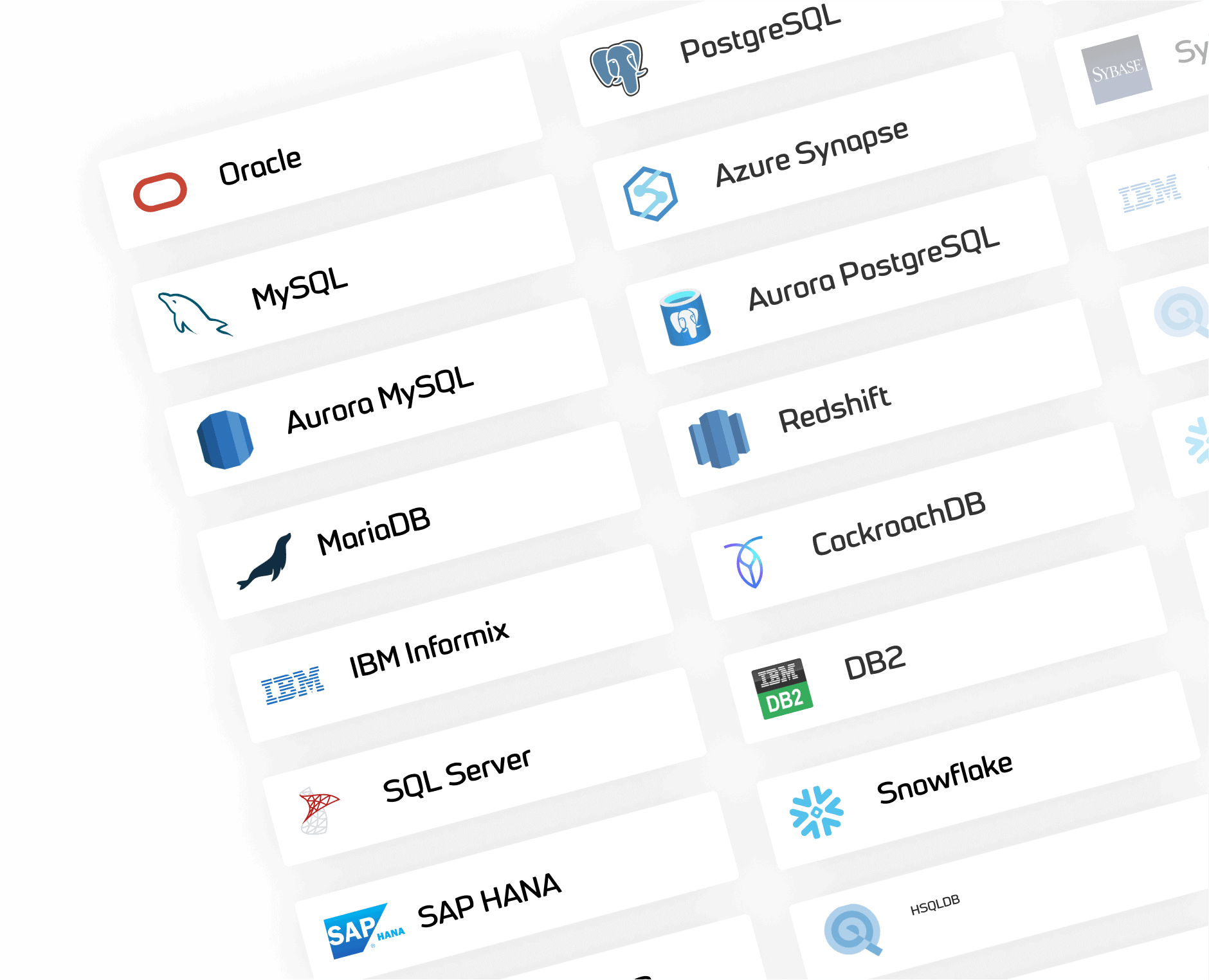
Connect, learn, and grow
We have a commitment to the community that extends beyond technical articles to real, tangible support for grassroots activity: be it in our comments section or at conferences, user group events, and so on.
Join the conversation by liking, sharing and commenting on our posts.
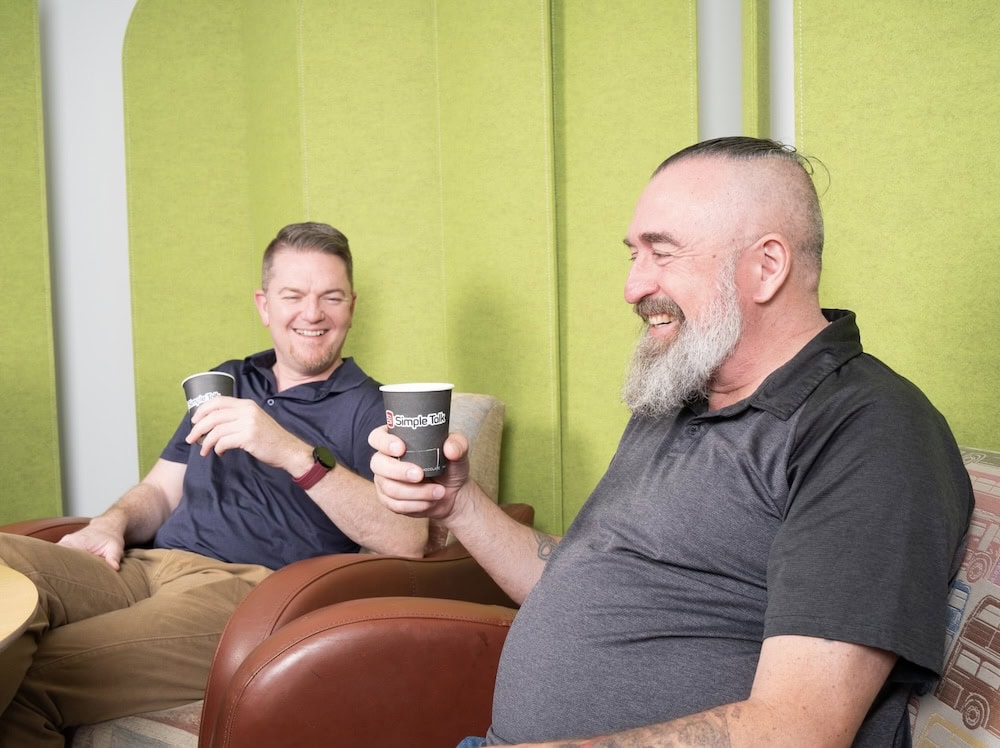
Check out our podcast: Simple Talks
Simple Talks features hosts Louis Davidson, Steve Jones, Grant Fritchey, Ryan Booz and Kellyn Gorman as they discuss technology adoption, career stories, industry challenges and more. From database management and DevOps, to data security and programming techniques, it’s a must-listen for tech industry professionals and enthusiasts.
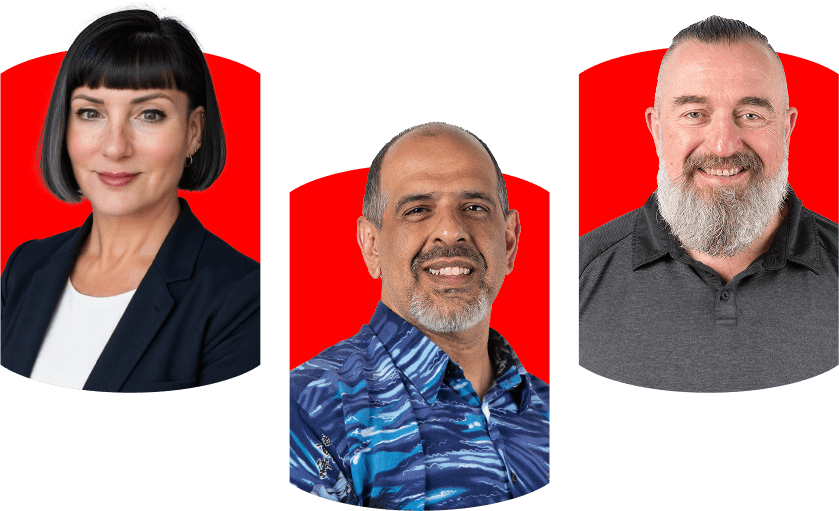
Contributions from the Redgate Advocates and the Simple Talk community
The blog wouldn’t exist without input from the community – after all, our authors are made up of technology and data professionals from all over the world. Simple Talk aims to provide high-quality, accurate technical content which has been peer-reviewed and written by technology professionals, not AI.
Simple Talk is brought to you by Redgate Software
Redgate creates ingeniously simple software to help organizations and professionals get the most value out of any database, anywhere, through the provision of end-to-end Database DevOps.
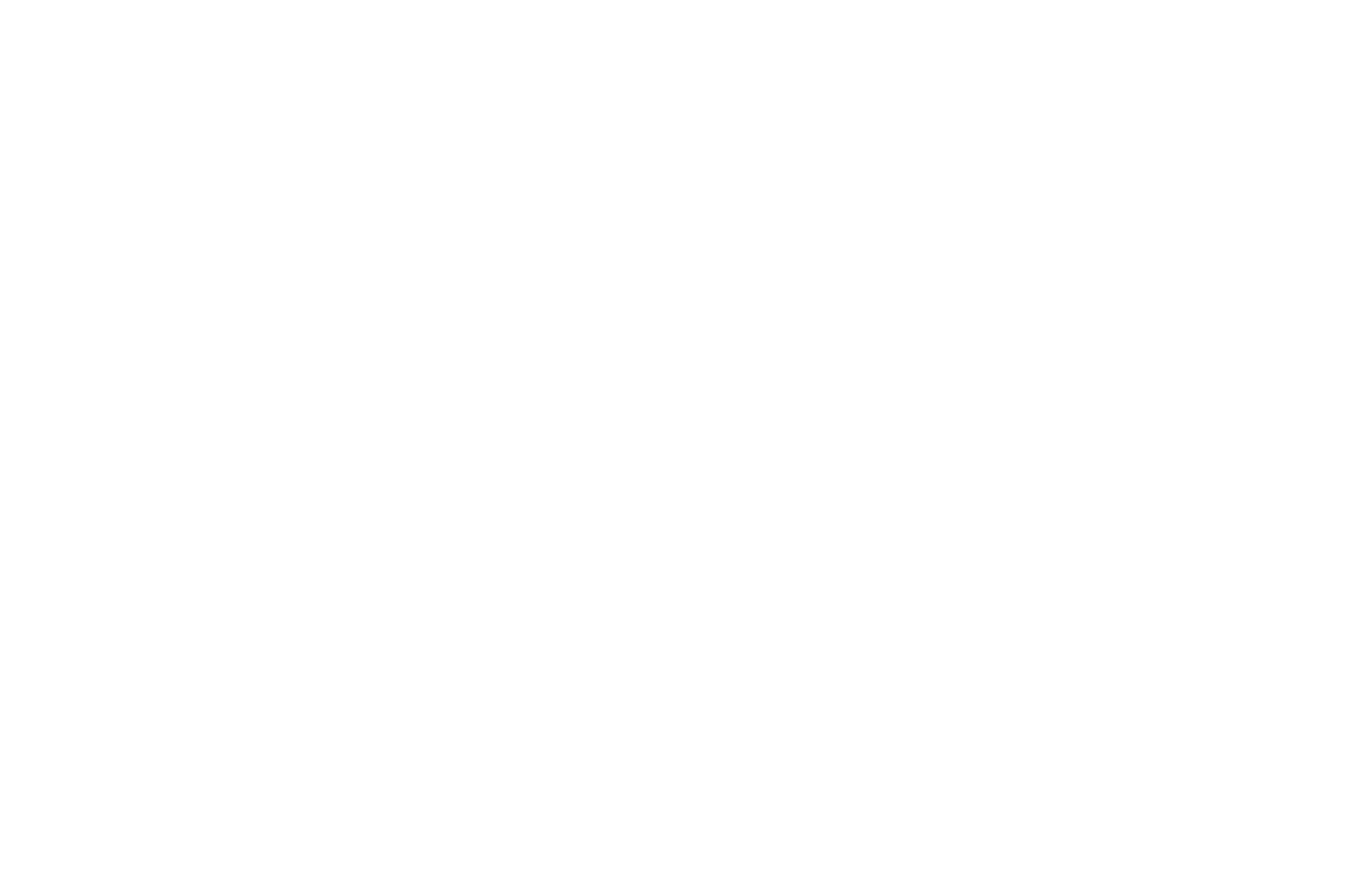